TLDR: We’re at an inflection point where it’s now economically viable to measure and make available data that was previously latent (energy, oceans, soil, air, satellite imagery). Bringing climate-relevant data online will unlock new innovative use cases including AI applications.
Knowledge is power. It’s obtained through access to information, but also requires knowing what to do with it. We’re in the midst of an ever-evolving AI revolution with mind-bending products like ChatGPT and Midjourney, but what’s going on under-the-hood? I promise there’s a climate angle to all this, but first let’s look at AI-generated images of Deadpool.
Back in March, when Adobe launched their own AI image generator, Jim Fan put together a thread comparing Adobe Firefly to Midjourney:
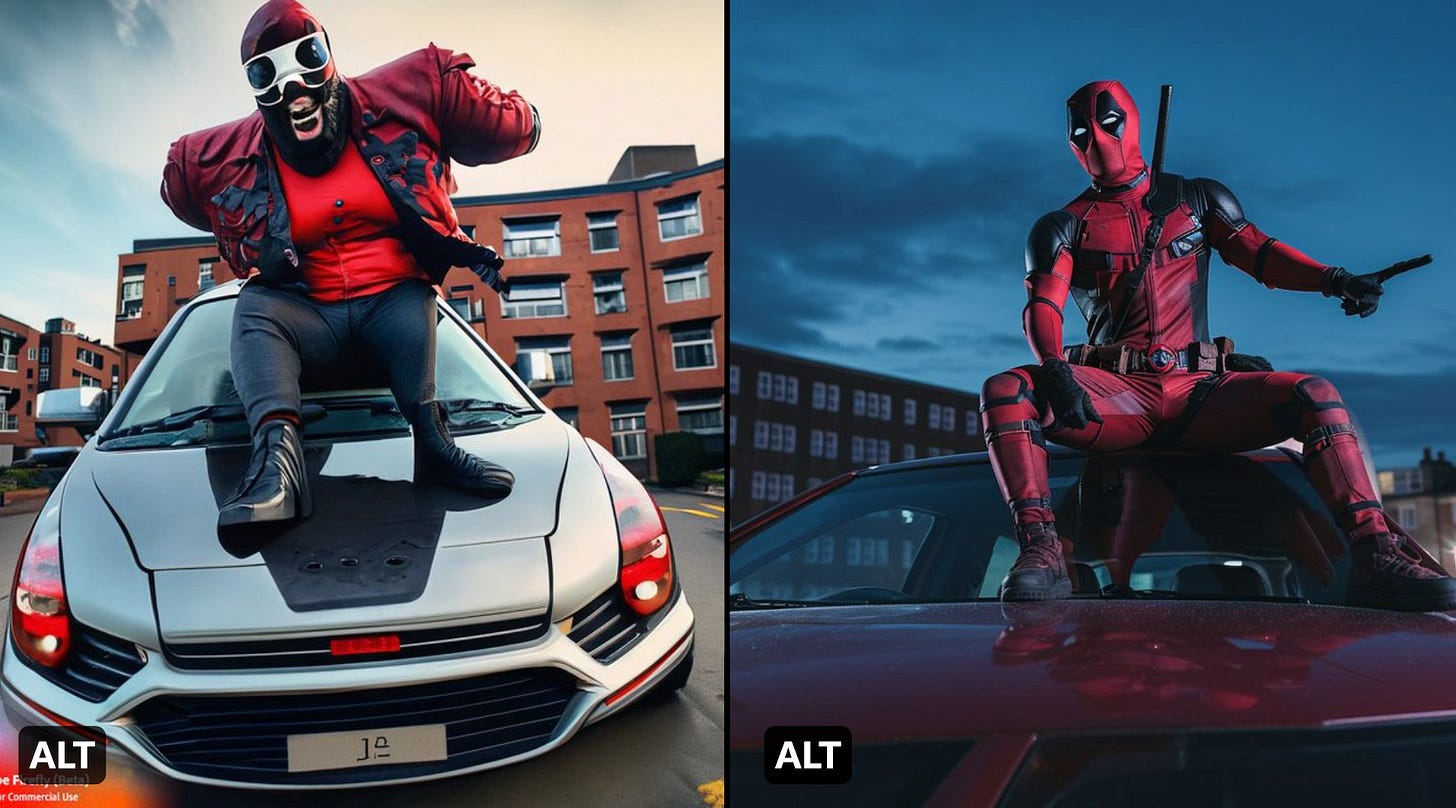
As you can see, the differences are clear. Without a trained eye, Midjourney’s Deadpool (right) looks indistinguishable from Marvel’s own studio. On the other hand, Adobe’s… is just not even close. There’s a reason for all this.
In AI, the model is only as good as the training set. The world’s leading AI engineers could team up to develop the best algorithm, but if they don’t have access to the relevant data, then it’s pointless. This explains why Adobe, a $175B company with 26,000 employees, couldn’t come close to Midjourney, a 10 person startup. While Adobe relied solely on fully licensed images, Midjourney did not. Instead, they entered a gray area by training their models on millions of publicly available images.
Across data formats (text, audio, image, video) and functions (sales, marketing, education, design), multiple AI startups charge towards the next frontier. However, this is just the tip of the iceberg. Current AI applications were trained on the easiest to obtain data sets (online and publicly available text and images). There remains a plethora of unexplored data and therefore, undervalued use cases. In some instances, such as in healthcare, sensitive patient information is inaccessible. In other cases, the data isn’t even being measured, as we’ll see is the case in many areas of climate relevancy. That’s starting to change as we now have sufficient incentives to measure our energy usage, ocean waters, soil, air, and landscapes via satellite.
The potential value of data isn’t evenly distributed
As consumers, we experience our data being measured, analyzed, and transformed back to us in delightful experiences everyday. Spotify has access to our listening behavior and offers personalized recommendations and a shuffle button that seems too good to be truly randomized. We improve our health with glucose monitoring from Levels and sleep tracking with Oura. Even the contentious domain of advertising provides some positive value to us. I’d much rather see relevant hyper-personalized Instagram ads from eCommerce brands than the past days of watching generic TV ads for antidepressants and osteoporosis meds.
As consumers, there are clear quality-of-life upgrades (and potential harm) that our data unlocks, but, when it comes to energy and the environment, we’ve been flying completely in the dark. Companies like Facebook and TikTok know so much about us, yet we know so little about our environment. As momentum builds to solve the environmental crisis, we’ll measure and expose new data types across ocean water, soil, air, energy usage, and satellite imagery. How might we use these data to curb emissions? And what other use cases will get unlocked as a byproduct? That’s what I set out to investigate.
The 5 elements of climate-relevant data
Throughout the history of civilization, humans have endured every major life-threatening crisis from pandemics to world wars. The optimist in me believes that at every existential junction, we embrace human nature and figure out how to survive. As the climate crisis has worsened, the incentives and motivation to address it have also risen (albeit not nearly enough). Given this complex, multifaceted problem affects every sector in the global economy, there is no “climate industry” or climate-specific data. Although greenhouse gas emissions (measured in gigatons) are climate-specific, myopically relying on carbon emissions as the sole metric fails to recognize how interconnected these systems are and how much signal upstream data can provide.
Rather than the binary labeling of climate or non-climate, I find it useful to view data through the lens of a climate relevancy spectrum since just about anything can be framed as climate-related. For example, using video calls instead of business-class flights and in-person meetings saves emissions, but we don’t necessarily view Zoom as a “climate company”. With GHG emissions established as the northstar metric in the climate community, I identified five additional data types that are climate-relevant and worth paying attention to: energy, water, soil, air, and satellite imagery. For each, there’s a mix of use cases that directly address the climate crisis, but also non-climate applications that will improve society in other substantial ways.
Energy 🔋
Several trends are playing out simultaneously. The proliferation of distributed energy resources (DERs) and subsequent increased demand for electricity will squeeze the already overloaded, centralized grid. Efforts to decarbonize the grid (without returning to the caveman era) require rapid electrification of buildings, manufacturing plants, and logistic networks. Electricity rates continue to rise and during bursts of peak demand we suffer from rolling blackouts or even worse, state-wide power outages that last several days. Strong demand to bring utility-scale renewables online balanced with stability has resulted in interconnection delays of months or even years. We need to integrate more renewables, beef up transmission lines, keep rates affordable, and avoid future disasters all at the same time. Measuring, unlocking, and then utilizing energy data will help us tackle these complex adaptive systems.
But first, we need to get the data
The first step to leveraging energy data is to actually get it. Until recently, the process to access energy consumption data was clunky and opaque. Even if it was just to simply look at your own home’s energy consumption. In 2021, Arcadia announced Arc, their API platform that enables developers to access rich utility data. Similar to how Plaid enables new ways to use money by connecting companies to consumer bank accounts, Arcadia enables energy use cases by connecting developers to utility energy consumption activity.
The API model is not the only approach. Australia-based Gridsight builds software for utility companies to better understand grid performance, proactively address safety issues, and verify DERs. In Europe, Gorilla is following a similar approach by aggregating and stitching together disparate data across smart meters, EVs, and IoT devices. By helping utilities understand energy traffic patterns like a moving heat map, they’re able to forecast demand more precisely and then price accordingly. These startups are able to facilitate information across different parties because they have root source access, down to the smart meter (the physical device that measures energy usage).
Use Cases
Once we’re able to actually measure and then responsibly expose energy data, a variety of use cases gets unlocked. Here are just a few examples:
Optimize EV charging rates by only charging the car when rates are low. The opportunity: The average EV owner in California overspends on charging by approximately $4,300 over five years.
Streamline the consultation and billing process for solar and storage installers by providing instant access to the homeowner’s energy consumption. Installers can use the current utility bill as a key input to design the appropriate solar panel system.
To meet regulatory requirements and sustainability requirements, companies need to know how much water, natural gas, and electricity they’re consuming. Having instant access to utility data can reduce a lot of headaches and manual paperwork when it comes to ESG reporting.
Demand response programs help stabilize the grid by incentivizing consumers and businesses to dampen demand during peak load times. Surfacing energy data insights to decision-makers is far more effective than shaming people or automatically cutting off their power.
As intelligent layers for energy data are created, the sheer volume of data will multiply and new opportunities will arise. With greater adoption for DERs and IoT devices, we’ll start to see the energy network look less like a strongly centralized grid and more like a large node (the grid) with many smaller nodes connected together. With vehicle-to-grid and home electrification picking up in momentum, new complexities such as bidirectional power flow will arise and the ability to measure, understand, and act on energy data will become even more important than it already is.
Further reading: Lessons from Plaid for a future energy unicorn
Oceans 🌊
Isn’t it crazy that we know more about outer space than our oceans? Ocean waters occupy 71% of Earth, yet 95% remains unexplored. When it comes to visualizing climate change, we see rising sea levels and that emaciated polar bear stranded on ice, but what else can we garner from the oceans? As it turns out, there are a few players today yearning to understand our oceans.
Sofar develops and deploys solar-powered buoys called Spotters. They float in the water and collect data on waves, currents, wind, and temperature. My personal experience with oceans is limited to surfing in them and observing from above on flights so I was surprised to learn that there’s significant enough variation in oceanic weather patterns to justify shipping companies paying for Sofar’s ocean intelligence platform to optimize their routes, saving 3-5% in fuel costs.
The aquaculture industry also benefits from measuring oceans. By deploying Spotters on site, fish farms are able to closely monitor fluctuations across a variety of data: conductivity, salinity, temperature, depth, eulerian marine currents, density, fluorescence and turbidity. These insights could also be useful for scouting potential aquafarm locations which require proximity to the proper logistics networks.
Another startup, Saildrone, is also tackling ocean data, but with larger unmanned autonomous vehicles and a different business model; they’re going after the surprisingly large $6B weather forecasting market. Did you know: one of the OGs in the space, Accuweather, generates $100M in revenue per year serving railroads, amusement parks, police departments, and Starbucks just to name a few. With a native weather app on every phone, we take for granted how valuable an accurate forecast can be. From my own experience of relying on the forecasts of Surfline for surfing and OpenSnow for skiing, I’ve seen firsthand how far we still have to go. Today’s forecasts are only accurate and reliable if you look 1-2 days out.
Recognizing the opportunity, Saildrone is approaching the problem of weather forecasting with a novel approach. They’re creating a proprietary data set measured by their robots in vast oceans that most weather stations can’t access. Not only are they logging previously undiscovered data, they’re also positioning in high value locations since most weather forms over the oceans. With its unique data set and accompanying forecasting models, they’re able to serve non-climate customers like sports teams, insurance companies and hedge funds, as well as climate-relevant government agencies like the National Oceanic and Atmospheric Administration and NASA. Saildrone exemplifies one of my core climate beliefs: the transition to a decarbonized economy will result in the convergence of pro-climate and pro-business products and companies.
Bringing new data types online has second-order effects that we’re only just starting to understand. With their capabilities, Sofar and Saildrone are pursuing their primary revenue opportunities in the freight and weather forecasting industries, but they’re also accelerating other climate businesses. For example, there’s currently a lot of hype around kelp’s potential to transform the food, textiles, and carbon market spaces. Kelp production will need to increase by orders of magnitude and understanding the aquafarms that it grows in will be vital. Kelp is just one example of a nascent climate solution leveraging climate-relevant data. In years to come, we’ll see more climate businesses that build off this newly unlocked data just how Uber depended on the smartphone’s GPS and Figma utilized the browser rendering technology WebGL.
Soil 🕳️
Nearly 50% of habitable land is used for food production (including land for animal feed and grazing), yet we know very little about the foundation for all agriculture: soil. In addition, there are some worrisome trends converging: 25% of agricultural land is highly degraded, soil degradation is reducing arable land in China and Africa, and population increase in developing countries is driving up demand for meat (and its associated land use). On the bright side, it’s estimated that soil could sequester up over 10% of all anthropogenic emissions in 25 years or over a billion tons of carbon each year.
The main constraint today is the cost to accurately measure and analyze soil organic carbon (SOC). Robust measurement, reporting, and verification (MRV) previously required manually digging up soil on-site and then mailing it to a lab where it would be incinerated to determine carbon density. This back-and-forth laborious process resulted in prohibitive costs to scale soil carbon sequestration. With cloud computing, satellite imagery, and sensors rapidly becoming more accessible, there’s a new crop (pun intended) of startups that are tackling the MRV component of the soil carbon market.
With Yard Stick’s handheld hardware device, users are able to collect and instantly measure the soil organic carbon. They couple the physical measurement device with an analytics dashboard that allows the user to map out soil carbon data and then share relevant insights with stakeholders (such as the businesses that are paying for carbon credits).
Taking a different approach, Perennial zooms out by combining soil sampling with satellite imagery and modeling techniques they claim is a lighter-weight approach than pure sampling. By leveraging a modeling approach that gets better with each incremental sample, they need fewer samples which results in faster, cheaper soil carbon measurement.
Although soil MRV is being accelerated by the carbon markets, understanding the underlying data will extend to all industries that rely on healthy soil to thrive, namely agriculture. One way to solve the declining collapse of arable land is to go upwards and denser with vertical farming, but another viable approach is to improve the efficiency of our existing farmlands, which requires this initial diagnostic layer. Whether it’s for carbon sequestration or simply improving crop yield performance, understanding the soil we stand on and live off of is a prerequisite worth building.
Air 💨
So far, we’ve been mostly discussing climate under the assumption that we’ll have enough time for the deployment and adoption of these solutions. I’m far from a doomer, but unfortunately, many signs point to more forest fires and droughts. Over the coming years, harsher conditions like forest fire season in California will require every household to stock up n95 masks and get an air purifier. As climate adaptation and mitigation becomes increasingly necessary, we’ll see more companies emerge to help bridge the gap between now and a future decarbonized world.
Companies like Clarity, Breezometer, Aclima, and Airly are all tackling air quality and greenhouse gas monitoring each in their own way. By leveraging sensor data and combining partner data aggregated from APIs, these startups are able to not only monitor air quality in one location, but also stitch together nodes to display and forecast trends.
Organizations like government agencies, schools, and relevant businesses like HVAC contractors are keen to leverage real-time air quality data to keep communities safe and healthy. Also, in order to form a comprehensive pulse on global GHG emissions, we’ll need a network of measurement devices that can paint the whole picture. Lastly, I wonder: What is the intersection of direct air capture and air quality monitoring? Could DAC technology be repurposed to also suck out particulates and pollutants?
Satellite Imagery 🛰️
The final element is both a data type and a technology. While the literal understanding is the physical equipment, the true value in satellites is derived from capturing images of relevant landscapes. For context, satellites have come down in cost from what was once $400M per unit to being manufactured in factories for less than $1M. As a result, the number of satellites being launched into space is exploding exponentially:
With each satellite equipped with imaging and Lidar, startups are leveraging satellite data in a variety of ways, including monitoring our forests. Pachama monitors forests, estimates carbon storage capacity, but also sells carbon offsets in the form of forests. The buyers of these carbon credits are large enterprises like Shopify, Salesforce, and Workday and they’ve all made public sustainability commitments to their shareholders. I’m sure the leaders of these companies have the right intentions, but the incentives are structured to try and get the most bang (carbon credits) for your buck. Even with satellite data augmented by remote sensing, drone-captured data, and trained by ML models, it's still impossible to be 100% accurate with the true carbon storage, so the question arises: how accurate is it currently? Perhaps this question of validity isn't being asked because it would nullify both the verifier and the buyer’s reputation. Take a step back from climate and ask how is it possible to verify and sell the thing you’re verifying without the waters getting muddied. Like Charlie Munger said, “Show me the incentive, and I will show you the outcome.” Even though I’m questioning one way that satellite imagery can be used, I still believe there is significant opportunity to leverage satellite data for climate use cases.
One day we might reach a point where energy becomes so cheap that we can manufacture and deploy autonomous drones to measure every tree on the planet, but we’re far from that fantasy. Today, I believe the opportunity is in areas where satellite imagery is still a step-function improvement from the status quo, but doesn’t demand the precision that carbon credits should. For example, Vibrant Planet is building a platform for planners and policy makers to map out forests and identify areas for reforestation.
Through an accessible UI powered by satellite imagery, Vibrant Planet is able to facilitate fire districts, the USDA Forest Service, state agencies, and local watershed groups to collaborate on forest management.
Similarly, Agreena services the agriculture MRV industry with remote sensing by measuring cover crops, tillage, and crop rotation. Monitoring these farming phases is critical for regenerative practices to restore soil and ecosystem health.
In the realm of mitigation and adaptation, there’s Floodbase, a startup leveraging satellite imagery, weather data, and on-the-ground sensors to provide flood-related insights and insurance.
Although I’m still hesitant on satellite imagery’s ability to precisely measure granular data like carbon storage potential, there will surely be more climate-relevant use cases as the number of deployed satellites continues to exponentially grow, even if they orbit at a lower data granularity.
Summing it all up
Climate-relevant data that was previously unavailable due to impractical economics is starting to become more accessible. As data across energy, ocean, soil, air, and satellite imagery comes online, there will be a new set of businesses that utilize this previously untapped data. It’s hard to know exactly how it all unfolds, but by continuing to ask "what if?", we’ll see how climate-relevant data can be leveraged, perhaps in ways that may even rival generative AI transforming media and culture.